Di Huang
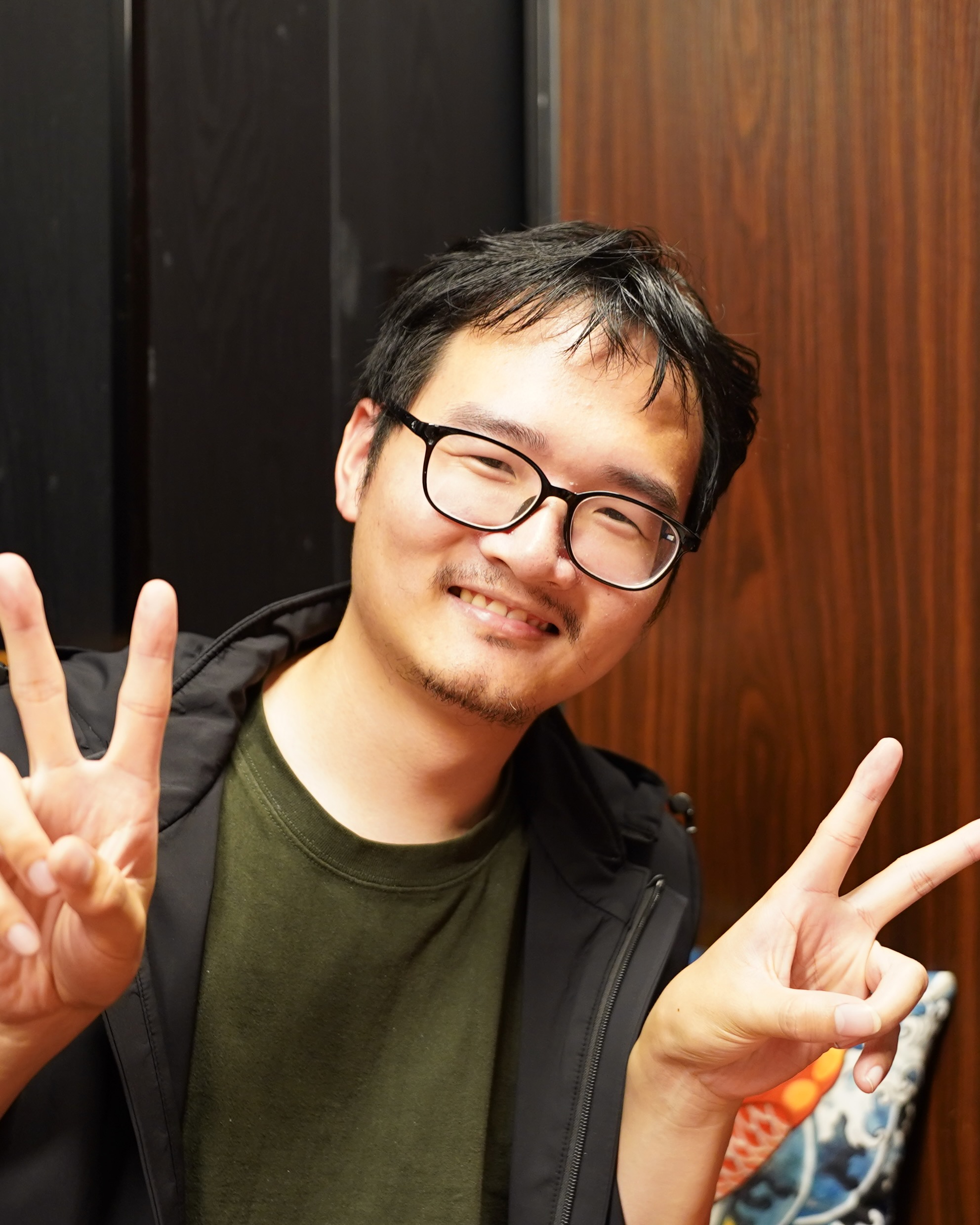
I am a researcher at the Shanghai AI Laboratory , where I collaborate with a team on advanced AI research, with a particular emphasis on 3D Vision. Concurrently, I am a Ph.D. student at The University of Sydney, working under the guidance of Wanli Ouyang on computer vision topics.
Previously, I earned my B.E. degree from Zhejiang University and had the privilege of graduating with honors from Chu Kochen Honors College. During my time there, I had the valuable experience of working with Xiaowei Zhou at the ZJU-3DV Lab.
Dear prospective students: We are looking for self-motivated students and research interns, please drop me an email if you are interested in working with us (for 3D Vision & Generative AI).
news
Mar 3, 2024 | Talks of 2023 have been uploaded. Talks of 2024 will start soon. |
---|---|
Feb 27, 2024 | One paper accepted to CVPR 2024. |
Dec 4, 2023 | New personal website launched. |